-
Cisco is using Ethernet to democratize AI usage beyond the hyperscaler elites
-
The demands of AI GPU computing demand new approaches in data centers
-
Company sees AI for CSPs and enterprises as key growth opportunities
For four decades, the folks that manage computer networks have been playing a game of whack-a-mole with performance bottlenecks in two locations: CPUs, and the networks that connect them.
The task is complicated by the problem’s refusal to stay in one place; each time a new and faster generation of processor or network rolls up, the chokepoint keeps jumping (ping, pong) between the two.
The arrival of Nvidia’s boss-level GPU AI chip has shifted the bottleneck squarely back onto the network side of the equation. That’s forcing a rethink of how data centers should be architected to handle the outlandish power requirements and data storage needs of the parallel data processing used in artificial intelligence (AI) applications.
Cisco Systems thinks Ethernet is the answer. The vendor developed an integrated portfolio of data center technology based on the world’s most established LAN technology, designed to commoditize AI data center infrastructure beyond the hyperscaler elite and allow enterprises and communications service providers (CSPs) to take part in the great AI migration.
RELATED
Hyperscalers want to replace Infiniband with Ethernet – Here’s why
Building AI data centers has so far been something only hyperscalers had the means and the wherewithal to undertake. Meta, for instance, boasts that it already has two clusters outfitted with over 24,000 Nvidia H100 GPUs (by the end of this year it plans to have 350,000 of the processors powering its AI infrastructure).
Which is nice for Meta. But like others of its ilk, the combination of Meta’s profitability (boosted by its single-minded commitment not to pay pesky taxes like the rest of us) means it has virtually unlimited money and resources to custom-build AI data centers from the ground up.
Enterprises and CSPs are in a different economic boat altogether – a steady tugboat putting along next to the hyperscalers’ cigarette boats. They need the potential revenue and cost-saving benefits of AI, but they need to do so according to a sensical business plan.
That’s where Cisco says it can help.
So what is it offering? Like just about everyone else in the industry, Cisco has a collaboration with AI chip leader Nvidia, which has been handing out JVs to vendors in 2024 like Oprah Winfrey gives out cars.
The deal marries Nvidia’s GPU technology to Cisco’s data center technology, and then uses Cisco’s legendary enterprise sales channel to bring the combo to the wider market. Clever.
Ethernet vs. InfiniBand
Cisco’s choice of Ethernet as the network infrastructure to support AI in the data center, rather than InfiniBand, is mildly controversial, depending on who you talk to.
At first glance, InfiniBand seems like a better fit for AI service, featuring an architecture with a slew of features designed specifically to provide a high-speed, low-latency, highly reliable interconnect between computers undertaking data-intensive processing tasks.
In reality, Ethernet has spent four decades vanquishing all manner of competing network standards – from Token Ring, to ATM, to FDDI, to Fibre Channel. That’s partly because it is so well established with a vast knowledge base, but also because it has been continually upgraded and modified over the years (most recently to support non-blocking architectures that eliminate the packet loss that can interfere with AI apps).
In any case, arguing over which high speed LAN standard to use is a very 1990’s thing to do. These days the action, and the money, has moved up into the application layer, and network architects pondering which interconnect to use can rest assured that no one ever got fired for buying Ethernet.
Cart, meet horse
Amidst all the hoopla and hype about artificial intelligence it’s easy to forget that — like any computing technology — it needs a network. Without the right data center infrastructure it’s like a horse and cart, without the horse. If Cisco can successfully open a path for enterprises and CSPs to deploy AI in data centers at an affordable price point it changes the whole dynamic of the AI market itself, because these new entrants will use their facilities to develop new classes of AI apps and services tailored to their unique business cases, including within vertical industries like finance or transportation.
I talked to Kevin Wollenweber, SVP and GM of Cisco’s Data Center and Provider Connectivity organization about its AI data center strategy.
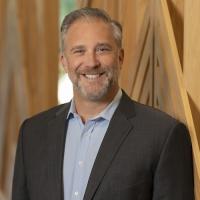
His comments were refreshingly pragmatic – a breath of minty fresh air in a market currently overpopulated with companies using the present tense to talk about AI capabilities residing somewhere in an undefined future.
Here’s what Kevin had to say when we spoke this week.
Steve Saunders: 2024 seems to be the year of AI?
Kevin Wollenweber: This is definitely the year that AI has exploded on the scene, but we’ve been building large AI infrastructure for a while. We have customers with reasonable size AI clusters today. Our goal is to build optimized Ethernet-based infrastructure for connecting AI based compute. We've been making heavy investments in silicon and switching technologies for decades, and as we move to 800 gig, and eventually 1.6 terabytes, we view the investments that we've made in optics technologies as critical to the way AI infrastructure evolves over the next few years.
Saunders: How do you see the AI market changing?
Wollenweber: Most of the GPU spend over the last couple of years has been with the hyperscalers. For Cisco, the next wave of AI lies with taking that compute capability and bringing it into the service providers and the enterprises in a way that’s easy to deploy.
Building a large Ethernet fabric to connect GPUs at scale requires intelligence at the operational level so, with the Nvidia deal, we’re providing both our optics and networking solutions, but also our orchestration and management layer.
Saunders: Why are Cisco and Nvidia a good match?
Wollenweber: Nvidia started largely as an engineering company, with amazing technology, and they have a very high-touch relationship with the hyperscalers. We see the large enterprise market as the space where this marriage is successful for both sides. Cisco has an incredible reach into the enterprise, so we deliver that, but we also bring our networking expertise in [things like] automation, orchestration, and telemetry.
There’s much more to this deal than putting their GPUs into servers and reselling their technology. We're undertaking engineering co-development with them. The first output of that today are Cisco Validated Designs, or CVDs, which are jointly validated reference architectures or blueprints that the enterprise customer can use to make it simple to deploy and manage a large scalable fabric for connecting GPUs in a wide array of use cases.
Over time, what you’ll see is solution-based engineering level deliverables, which go beyond connecting AI servers into a fabric, to allow our orchestration to help with things like job scheduling. Also, this type of development is where the convergence of AI processing and networking starts to get really interesting.
Saunders: Will AI spread into vertical industries?
Wollenweber: That’s part of the next wave of AI that I'm talking about, and this partnership will take AI into those verticals, beginning with financial services. I'm really excited to see what kinds of actual workloads people are running in those markets and drive deeper into them.
Saunders: Why Ethernet and not InfiniBand?
Wollenweber: Meta has built two 24,000 GPU clusters, one with InfiniBand, and one with Ethernet, and they’ve shown that by tuning Ethernet they're able to get consistent performance, and so they're now training LLAMA 3 [Meta’s autoregressive large language model] on a massive Ethernet-based cluster.
When I look at the history of Ethernet; the massive investment in it; the pace of innovation that we’re driving; it’s my view that it’s the right train to ride. We’re doing tons of work around efficiency, and the Ultra Ethernet Consortium is pushing it forward as well.
Then, when you look at the convergence of GPU-based compute, obviously it doesn't work without data storage, so we need to answer the question of how do we bring storage into this equation? Storage across [Ethernet] fabrics is a well- known technology. And so if you can build these scalable non-blocking fabrics, and then drive all of these technologies across them, then you have all the right pieces to develop an extremely efficient data connectivity and information sharing network.
RELATED
Data storage gets spicy with help from AI
Saunders: What do you see happening further out in the future?
Wollenweber: There's going to be some interesting advancements in optical, analogous to the way we have transformed transport networks by taking 400 gig pluggables that can be configured to run across multiple wavelengths and putting them directly into the routing devices. Once you have that coherent interface in the data center, there are some interesting things you can do from an optical perspective.
Saunders: Eventually it's just going to be optical direct to the CPU, or GPU, isn't it?
Wollenweber: At some point that will be the strategy. They’re already getting closer together, and as speeds go up, eventually we're going to have to move this into that optics domain, but when and where that happens is still an open question.
Saunders: People are talking about building bigger and bigger data centers, but doesn’t it make more sense to distribute the data and the processing to the edge of the network?
Wollenweber: Well, this is why this trend to move AI into the enterprise itself is so interesting. People are planning what they want to do and working with data scientists on how they can run AI at scale. And if you have large workloads, and you want to run your GPUs at high efficiency, then I agree, doing that at the edge of the network at the customer premises makes sense. And Cisco’s whole goal here is to make it easier for enterprises to be able to do things like that.