- Cloud hyperscalers and Elon Musk are racing to build data centers capable of training AI models
- These so-called AI factories cost billions to construct and have monthly power bills in the millions of dollars
- But they run the risk of becoming obsolete in the coming years as workloads shift to the edge
You probably heard about it in the news. Elon Musk is working with Dell and Nvidia to build a massive data center in Memphis, Tennessee to help train a new artificial intelligence (AI) model for Musk’s xAI venture. Musk, of course, isn’t the only one investing heavily in data centers for AI.
But there’s one key question not enough folks seem to be asking: could all these billion-dollar investments end up obsolete in a few years’ time?
“That’s the gamble,” analyst Jack Gold told Fierce.
A firm number for Musk’s so-called Gigafactory of Compute hasn’t been disclosed. Ted Townsend, president and CEO of the Greater Memphis Chamber, said only during a press conference the AI factory will represent “the city’s largest multi-billion-dollar capital investment” in Memphis history.
But Gold, president and principal analyst at J. Gold Associates, ran the numbers. His estimate? Assuming the factory includes 100,000 Nvidia H100 GPUs as Musk has stated, Gold calculated the machine cost alone for the factory is probably in the realm of $3.75 billion.
Then there’s the electricity cost for those machines, which Gold estimated will run anywhere from $3.96 million (at 50% utilization) to $7.92 million (at 100% utilization) per month. Those figures assume a total system requirement of around 1 kilowatt per machine (GPU plus networking, CPU controller, etc.) at a local electricity rate of around 11 cents per kilowatt hour.
So, Musk’s factory is not only pricey to build but also pricey to run. And Gold told Fierce his estimates don’t even include things like the cost of land, the building or cooling costs. (Musk, for the record, said the factory is using liquid cooling for the GPUs.)
With costs like these, Gold noted there’s only a handful of companies and people that can afford build a dedicated AI data center. And the question of whether they do so comes down to whether they believe they can get enough of a return on investment from the models they train there.
It seems many think they’ll get their money’s worth. Baron Fung, Senior Research Director at Dell’Oro Group, noted in a recent forecast that “AI has the potential to generate more than a trillion dollars in AI-related infrastructure spending in cloud and enterprise data centers over the next five years.” What’s more, Amazon, Meta, Microsoft and Google are expected to account for half of global data center capex as early as 2026.
Sea change
Right now, investing in AI data centers makes sense. After all, as Gold noted, AI compute utilization today is about 80% training and 20% inferencing. And those pumping cash into these AI factories are assuming they’re going to get the payback from training models and being on the bleeding edge of the technology’s development.
But Gold noted the compute equation is set to flip in the next three years or so, such that it is 80% inferencing and just 20% training. And as inferencing becomes the workload du jour, AI compute will move to the edge.
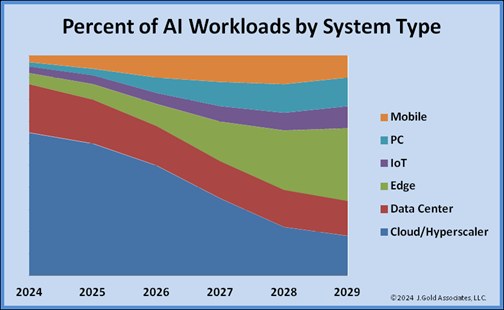
So, what happens then to the massive sprawling centralized data centers hyperscalers are building for training today?
“That’s a good question,” Gold said.
The first instinct might be to say ‘Oh, the hyperscalers can just put those GPUs to work on other tasks.’ But Gold noted there’s a problem with that theory.
“Turns out GPUs are not really very good at running databases or Oracle or SAP or whatever you’ve got to run,” Gold said. “CPUs are better at that.” What GPUs are good at, he said, is the parallel processing needed for AI.
So, it’s not immediately clear how these GPU resources will be reused or whether they might simply be scrapped and replaced with new compute better suited for future workloads. Meta, at least, appears to be thinking about the issue.
“When we think about sort of any given new data center project that we're constructing, we think about how we will use it over the life of the data center,” Meta CFO Susan Li said on the company’s Q2 2024 earnings call.
“There's sort of a whole host of use cases for the life of any individual data center ranging from gen AI training at its outset to potentially supporting genAI inference to being used for core ads and content ranking and recommendation and also thinking through the implications, too, of what kinds of servers we might use to support those different types of use cases,” she continued. “We're really doing that with both a long time horizon in mind, again, because of the long lead times in spinning up data centers, but also recognizing that there are multiple decision points in the lifetime of each data center.”