Industry Voices are op-eds from industry experts or analysts invited to contribute by Fierce staff. They do not represent the opinions of Fierce.
Open RAN is gaining momentum as major network operators such as AT&T, DoCoMo and Vodafone are making their plans more real, more immediate and more concrete.
But I have a word of warning for my friends in open RAN: What will happen when the top network vendors build AI enhancements into the RAN?
I have just finished a six-month project that culminated in a report on the capacity impact of AI on the RAN. Capacity is a major topic for our customers nowadays – mobile operators anticipate a “capacity gap” where demand in dense urban hot spots will outstrip their capacity in the 2027-2029 timeframe. Applying AI/ML techniques to the RAN can help to close that gap, with 20-30% improvement (see the report for details on uplink and downlink improvements).
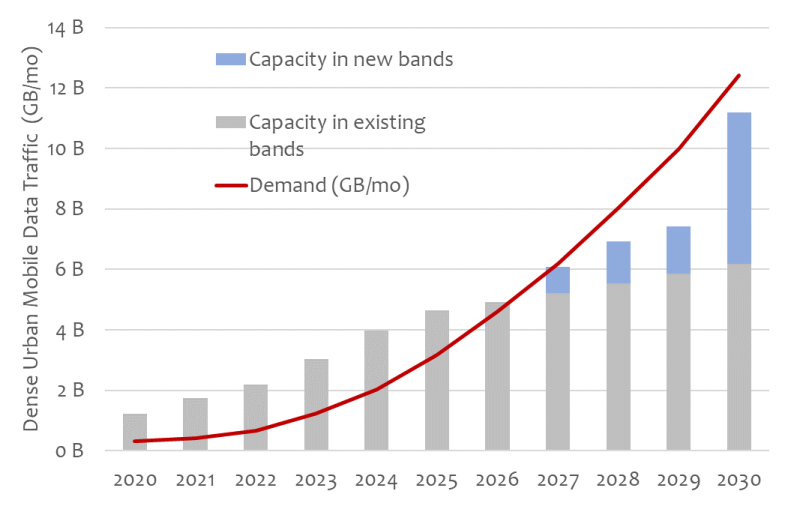
The above chart shows the capacity and demand in the 10% busiest cell sites in a major American network. The other 90% of sites won’t experience the same “capacity gap,” but in our dense urban centers, we’re going to see a shortfall of capacity in 2028 and 2029. The gray bars show capacity in existing bands and the blue bars show the new DoD band and an expected 6G band. Even if the spectrum-sharing limitations are light, there’s a problem here. AI/ML will be able to close this gap for significant numbers of sites.
AI will be one of the best investments that mobile operators ever make: a software upgrade that will cut Total Cost of Ownership by $0.08/GB, while preventing a churn-apocalypse from lack of capacity. The cost will be minimal, with some additional processor cores and a few GPUs.
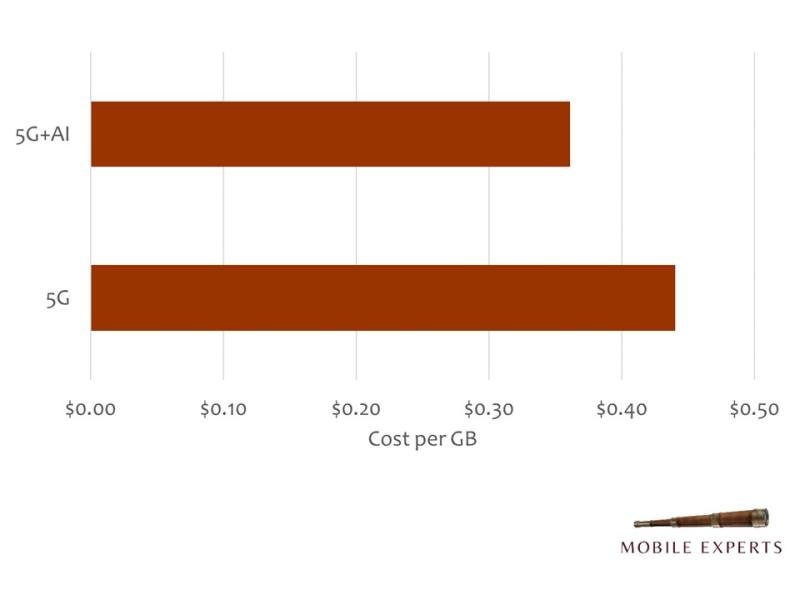
AI is not magic. It won’t stretch Shannon’s Law, as the hype says. But it will improve the average performance for the average user, which is the important outcome for the operators.
Data formats
Here’s the issue between AI and Open RAN: AI/ML models rely on hard data to refine and improve themselves. If an AI model is built and trained by Vendor A, using their own radio hardware, the real-time data in the AI/ML training set will be formatted in a very specific way. They may use I/Q samples or RF power measurements or a temperature sensor in the RU to make certain decisions. When a radio from Vendor B is substituted, all of the data will come in a different format. Can the model re-train itself with different data formats? We don’t know yet.
The O-RAN Alliance is working on this problem. The O-RAN Alliance Working Group 2 has a study item where they consider alternatives, and some vendors have suggested an excellent framework for sharing standardized data between RICs and SMO, such as RSRQ data and RB allocations. But this is not as rich or as real-time as individual RF measurements directly from each antenna element. The O-RAN spec has also not been updated since 2021. So the O-RAN approach can get some of the benefits, but not all of it.
Will Ericsson or Nokia work hard to solve the gap in performance? Each company will use its AI/ML model as a way to differentiate itself, giving its preferred hardware the best performance possible. If a major vendor can get 20% higher capacity performance using their own radio, operators will be tempted to use their incumbent vendor in the urban areas where capacity matters most.
In rural and suburban areas, the AI/ML models will prioritize energy efficiency instead of capacity. In those cases, open RAN vendor combinations are likely to work out well. The actions will take place at a slower pace (non-real time) and the decision to turn off radio channels or hardware will be based mostly on traffic data, not real-time RF measurements.
We live in interesting times. Multiple technology disruptions are happening at the same time. In this case, we have two technology disruptions that may disrupt each other. Be careful that you don’t get blindsided.
Joe Madden is principal analyst at Mobile Experts, a network of market and technology experts that analyze wireless markets.