Presenters:
Muninder Singh Sambi, Vice President, Product Management, Google Cloud Networking
Neelay Shah, Principal Software Architect, NVIDIA
Overview
As businesses increasingly operate in hybrid and multi-cloud environments, data is becoming more distributed. At the same time, enterprises are rapidly embracing multi-cloud for AI/ML and are planning to adopt generative AI within the next three years.
To meet the new requirements driven by AI while maintaining business agility, traditional technology is no longer sufficient—enterprise networks must be secured, streamlined, and scaled for AI applications.
Google Cloud offers the most comprehensive and efficient AI infrastructure, providing customers with unmatched scale, coherence, choice, and flexibility. Google Cloud Networking ensures low latency and high bandwidth for AI/ML traffic, while GKE and Model-as-a-Service endpoints enable seamless access to models across various environments. By leveraging these capabilities, enterprises can unlock the full potential of AI/ML, accelerating innovation and achieving tangible business outcomes.
Context
The presenters discussed AI-driven technology evolution and shared five key recommendations for serving AI applications.
Key Takeaways
Gen AI is driving a fundamental evolution in networking technology.
As more enterprises adopt Gen AI, GPU demand has skyrocketed, but with new technology comes new challenges. Traditional web applications and Gen AI applications have different traffic patterns that result in different network requirements.
Traditional web apps | Gen AI apps |
---|---|
|
|
To leverage the power of Gen AI requires fundamentally different technologies. Achieving AI use cases depends on advancements such as large, specialized compute infrastructure; a low-latency communication fabric; high-bandwidth data transfer; and more.
“In [AI] use cases, networking has to work. It is a significant enabler to help pave the way.”
- Muninder Singh Sambi, Google Cloud Networking
Figure 1: AI applications have different use cases from traditional applications
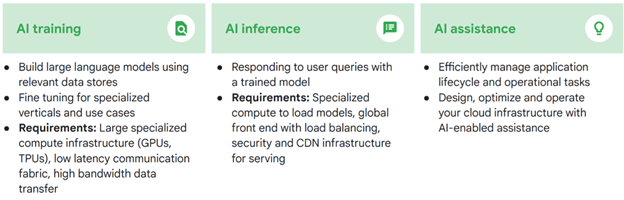
Google offers 5 key recommendations for managing the network for AI workloads.
Through its longtime partnership with fellow industry leader NVIDIA, Google has developed innovative technology to serve Gen AI applications, simplifying infrastructure to enable greater velocity and help meet constantly changing requirements.
The collaboration between Google and NVIDIA has not only yielded considerable advancements in AI technology, but also provided a wealth of experience from which Google has drawn five key recommendations for managing the network for AI workloads:
1. Establish a scalable network fabric built and optimized for AI/ML infrastructure
The four stages of the AI/ML pipeline are data ingestion, data preparation, model training, and inference. The underlying network infrastructure plays a critical role at each stage in ensuring performance, latency, and cost efficiency. A high-capacity, non-blocking data center network optimized for training is essential to optimizing job completion times when conducting model training.
“By optimizing the network infrastructure at each stage of the AI/ML data pipeline, enterprises can now achieve significant movement in overall model development and deployment efficiency, leading to faster time to market and better user experience.”
- Muninder Singh Sambi, Google Cloud Networking
Figure 2: A scalable network for Gen AI
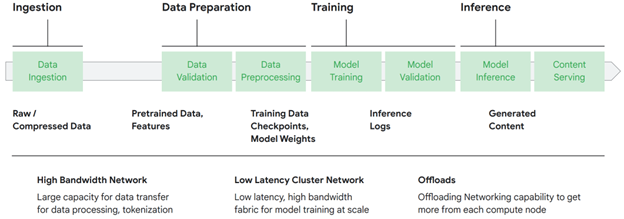
2. Train AI across clouds with the Google Cross-Cloud Network
Training and inferences use cases depend on data ingestion. However, the data must first be moved from different locations (e.g., on-premise, in other clouds) to the AL/ML cloud infrastructure—without compromising security. The Cross-Cloud Network provides low-latency, highly reliable, hybrid and multi-cloud connectivity—backed by SLAs—for moving data rapidly and securely.
Figure 3: Google Cross-Cloud Network enables AI training across clouds
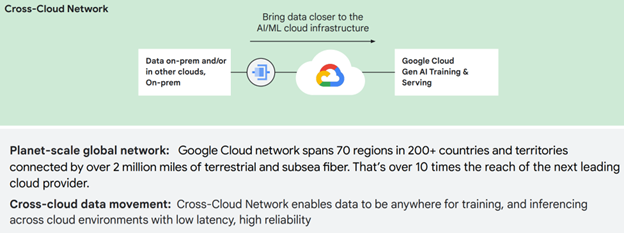
3. Secure AI workloads, data, and users.
Implementing comprehensive and pervasive security at every layer of the network is imperative for mitigating risk with Gen AI. Enterprises need high security efficacy and strong network controls that are simple to use. Google security solutions are built to provide security at scale in the constantly changing threat landscape, achieving a 20x greater efficacy than available alternatives for both data at rest and data in transit.
“No longer will there be any place where the data . . . or the model can be exposed, all the way from the application layer to GPU. Making [security] hardware-based is really exciting.”
- Neelay Shah, NVIDIA
Figure 4: Zero-trust security for workloads, data, and users is critical for AI applications
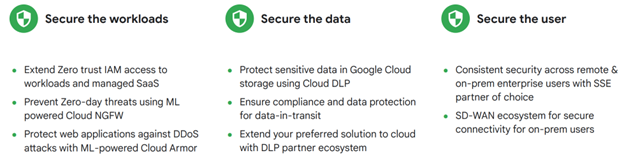
4. Optimize traffic management with load balancing for AI inference
The multimodal nature and varying request and response times of Gen AI inferencing workloads present unique challenges to network management. The traditional round-robin or utilization-based traffic management is not optimal for Gen AI-based inferences. Google Load Balancing solves this challenge by distributing traffic based on a customizable queue depth metric, lowering average and peak latency while also providing better GPU efficiency and lowering cost.
Figure 5: Google provides advanced load balancing for AI
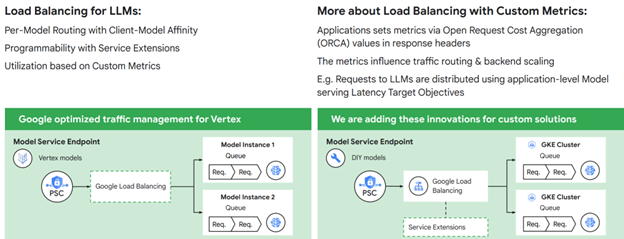
5. Operationalize with AI-powered Gemini Cloud Assist
Leverage the AI-powered Gemini Cloud Assist for operational efficiency. From design through build, Google Gemini will help optimize operations by providing guidance on best practices, such as reducing cost or improving security, offering troubleshooting advice, and more. Operationalizing the network with Gemini Cloud Assist accelerates the journey to the cloud.
Figure 6: Gemini Cloud Assist helps operationalize AI
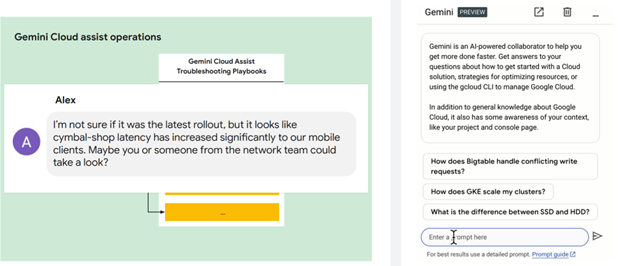
Learn more
Visit Google Cloud and Nvidia
Biographies
Muninder Singh Sambi
Muninder Singh Sambi is Vice President of Product Management for Networking at Google Cloud. He is responsible for setting the strategy, vision and investment strategy for Google Cloud Networking and Network Security products. Muninder carries over 25 years of leadership experience in product management and engineering within global high-technology environments. He has a proven track record for building next generation security, networking and as-a-service software products that have generated billions of dollars in revenue. A technical leader adept at managing product transitions, leading cross-functional, global product management teams, and outbound marketing teams. Muninder holds an Electronics and Communication degree and an MBA. He holds 8+ US patents in networking and security.
Neelay Shah
Neelay Shah is the principal software architect for NVIDIA Triton Inference Server and an AI solutions engineer. His focus is on enabling developers with a smooth transition from prototyping to high-performance production deployments at scale. Before joining NVIDIA, Neelay was a principal engineer at Intel leading open source projects for computer vision pipelines. He has a bachelor’s degree in computer science from Williams College and a master’s degree in computer science from UIUC.